Is Your Crystal Ball Cloudy? Why AI-Powered Forecasting Beats Traditional Methods
Discover why AI-powered forecasting is more accurate and reliable than traditional methods in our latest blog post. Learn how to clear up your cloudy crystal ball and make better business decisions with the help of artificial intelligence technology.
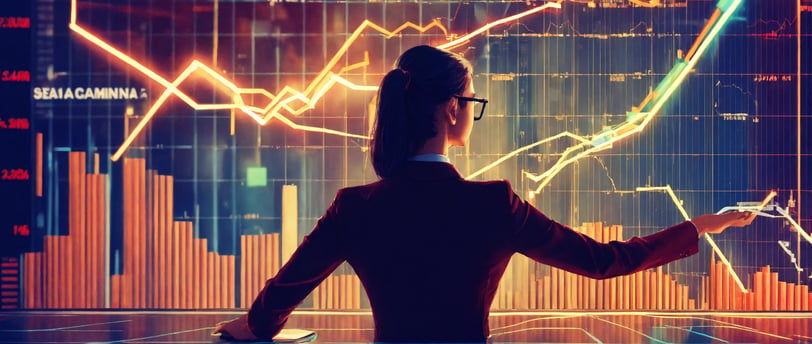
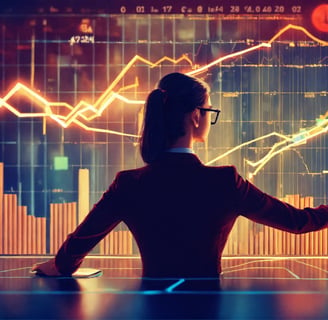
Overview of Forecasting Challenges
Forecasting in today's dynamic business landscape poses a multitude of challenges that organizations need to navigate effectively. The reliance on traditional forecasting methods often falls short in capturing the intricate patterns and complexities of data trends. As businesses seek more accurate and timely insights for decision-making, the limitations of conventional approaches become increasingly apparent. This is where AI-powered forecasting emerges as a compelling solution, offering advanced algorithms and machine learning capabilities to enhance predictive accuracy and efficiency.
The evolution of AI forecasting has reshaped how businesses approach planning and strategy development. By leveraging sophisticated algorithms and adaptive learning models, AI has the potential to revolutionize forecasting processes. However, integrating AI into forecasting practices requires a deep understanding of its capabilities and limitations, as well as a strategic mindset to harness its full potential. Striking the right balance between human expertise and AI intelligence is crucial to unlocking the true transformative power of AI forecasting in driving business success.
Understanding the Limitations of Traditional Forecasting Methods
Traditional forecasting methods have long served as the backbone of predictive analytics in countless industries, providing valuable insights into future trends. However, these methods often fall short when faced with the ever-changing dynamics of the modern business landscape. One key limitation is the reliance on historical data, which fails to account for unforeseen disruptions or emerging market trends. This can lead to inaccuracies in predictions and a lack of adaptability in forecasting models.
Another significant limitation of traditional forecasting methods is their inability to handle large volumes of complex data in real-time. In today's fast-paced environment, businesses require quick and accurate decision-making based on up-to-the-minute information. Traditional methods struggle to keep up with the speed and scale of data processing needed to provide timely insights. As a result, organizations may miss out on valuable opportunities or fail to anticipate potential risks, highlighting the need for more advanced and agile forecasting solutions.
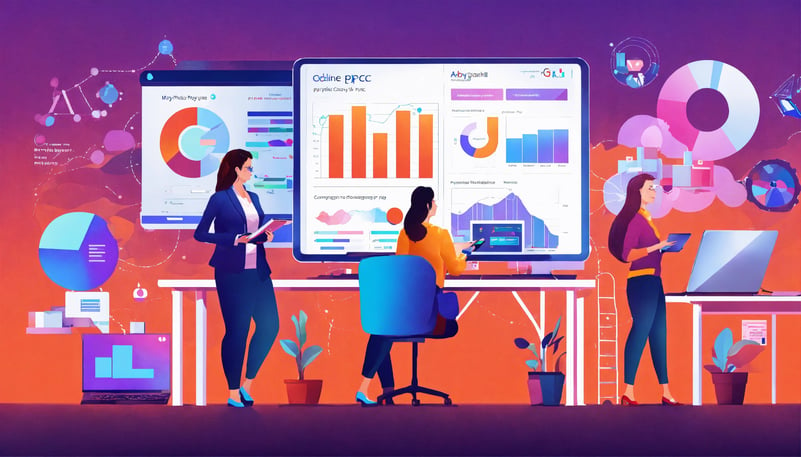
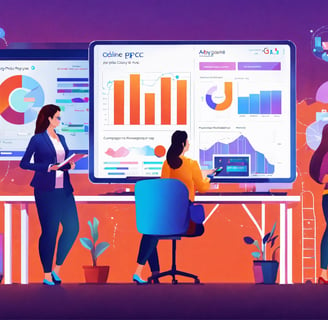
The Advantages of AI-Powered Forecasting
Artificial Intelligence (AI) has revolutionized the field of forecasting by offering advanced capabilities that traditional methods often lack. Machine learning algorithms, a subset of AI, play a fundamental role in enhancing prediction accuracy. These algorithms can analyze vast amounts of data swiftly, detecting complex patterns that human forecasters might overlook. This level of precision can significantly improve the reliability of forecasts across various industries, making AI-powered forecasting a valuable asset for businesses aiming to make informed decisions based on data-driven insights.
Moreover, AI-powered forecasting provides the advantage of adaptability and scalability. Unlike traditional methods that may struggle to cope with ever-changing data dynamics, machine learning models can continuously learn and adjust based on new information. This flexibility enables forecasts to remain up-to-date and reflective of the most current trends, offering businesses a competitive edge in an environment where agility and responsiveness are paramount. By leveraging AI in forecasting, organizations can harness the power of predictive analytics to optimize resource allocation, mitigate risks, and seize opportunities proactively.
How AI Improves Accuracy in Predictions
AI enhances prediction accuracy through its utilization of advanced forecasting techniques. By employing machine learning algorithms, AI systems can analyze vast amounts of data to identify patterns and trends that may not be readily apparent to human analysts. This level of data processing and pattern recognition enables AI to make more precise predictions based on complex relationships within the data, resulting in forecasts that are more reliable and accurate.
Furthermore, AI has the capability to continuously learn and adapt from new data inputs, allowing for dynamic adjustments to forecasting models in real-time. This adaptability ensures that AI-powered predictions remain up-to-date and reflective of the most recent data trends, enhancing the accuracy of forecasts even in rapidly changing environments. As a result, organizations leveraging AI for forecasting purposes can benefit from more reliable insights that are constantly refined and improved, ultimately leading to better decision-making and outcomes.
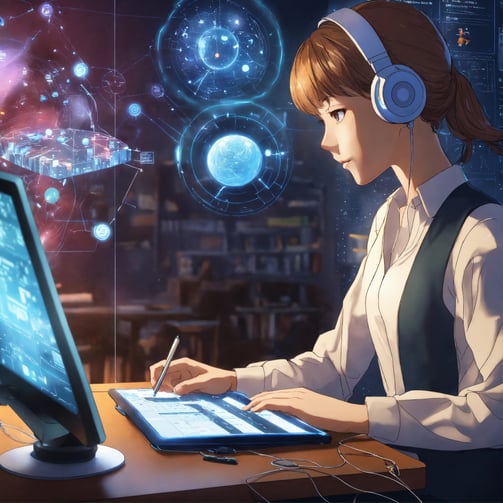
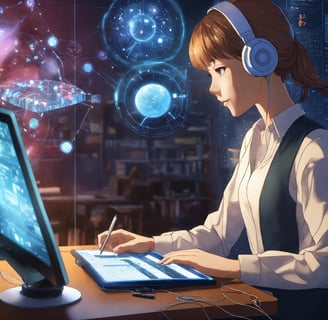
The Role of Data in AI-Powered Forecasting
Data-driven forecasting lies at the heart of AI-powered solutions, as the accuracy and relevance of predictions heavily rely on the quality and quantity of data available. High volumes of diverse, real-time data enable AI algorithms to identify patterns, trends, and anomalies that traditional methods may overlook. By analyzing historical data alongside current information, AI-powered forecasting can provide more nuanced insights into future scenarios, aiding businesses in making informed decisions.
The role of data in AI-powered forecasting extends beyond mere numbers; it encompasses the ability to extract meaningful insights from a sea of information. Through advanced analytics and machine learning, AI algorithms can detect correlations and dependencies within datasets that might not be apparent to human analysts. This granular level of data processing enables organizations to tailor their forecasting models to specific market conditions, customer behaviors, and operational factors, resulting in more precise and actionable predictions.
Benefits of Real-Time Data Analysis in Forecasting
Real-time data analysis holds paramount significance in the realm of forecasting. By harnessing the power of instantaneous data insights, organizations can bolster their forecasting accuracy to unprecedented levels. Leveraging real-time data provides a dynamic edge by allowing businesses to adapt swiftly to changing market trends and consumer behaviors. This agility in decision-making equips enterprises to stay ahead of the competition and make informed, proactive choices grounded in up-to-the-minute data.
Furthermore, the integration of real-time data analysis in forecasting processes enhances the precision of predictive models, leading to more reliable forecasts. Engaging with data streams as they occur enables businesses to detect patterns and anomalies swiftly, thereby optimizing strategies and mitigating risks promptly. By incorporating real-time data analysis into their forecasting framework, organizations can capitalize on opportunities with agility and finesse, securing a competitive advantage in today's fast-paced business landscape.
Case Studies Demonstrating AI Forecasting Success
In recent years, businesses across various industries have experienced significant improvements in forecasting accuracy by adopting AI-powered solutions over traditional methods. One such success story comes from a leading e-commerce company that transitioned from using outdated forecasting software to employing advanced AI algorithms. By leveraging AI's capabilities to analyze vast amounts of real-time data, the company was able to achieve a more precise prediction of consumer trends and inventory requirements. This shift from manual forecasting to AI-driven insights not only optimized their supply chain management but also resulted in substantial cost savings and increased customer satisfaction.
Similarly, a global telecom provider saw a remarkable transformation in their forecasting processes after switching to AI technology. By incorporating machine learning algorithms into their forecasting models, the company was able to anticipate subscriber churn rates with unprecedented accuracy. This level of foresight enabled them to proactively address customer retention strategies, leading to a significant reduction in churn and an uptick in subscriber loyalty. The success of these case studies underscores the pivotal role that AI plays in enhancing forecasting accuracy and strategic decision-making, highlighting the stark contrast between AI-powered forecasting and traditional methods in today's data-driven business landscape.
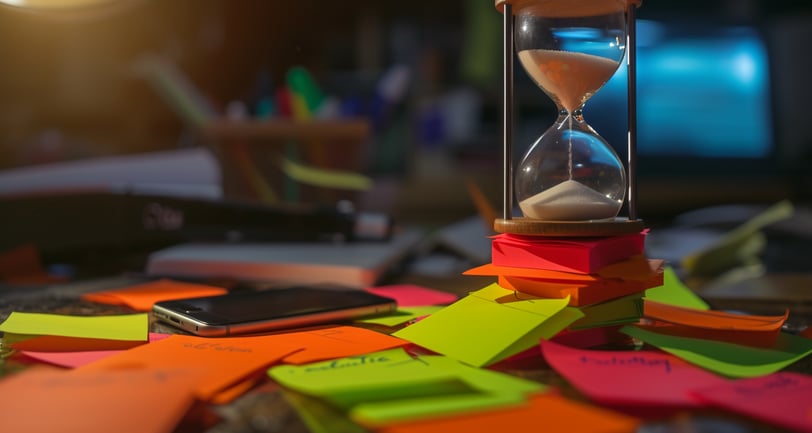
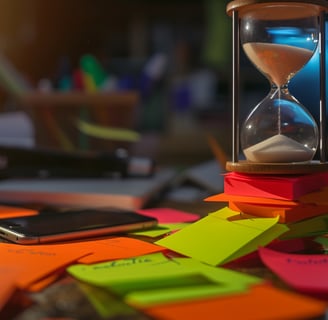
Implementing AI Forecasting in Your Business
To integrate AI forecasting successfully into your business operations, start by evaluating your current forecasting methods and identifying areas where AI-powered predictive models can bring added value. Consider the specific challenges your business faces in terms of forecasting accuracy, timeliness, and efficiency. This initial assessment will help pinpoint which aspects of your forecasting process can benefit most from the implementation of AI technologies.
Once you have identified the target areas for improvement, choose AI forecasting solutions that align with your business goals and requirements. Look for platforms that offer customizable predictive models tailored to your industry and business needs. Collaborate with AI experts or consultants to ensure a smooth integration process and provide training to your team to maximize the benefits of these advanced forecasting tools.
Considerations for Choosing an AI Forecasting Solution
When selecting an AI forecasting solution for your business, it is essential to consider the specific needs of your organization. Begin by assessing the complexity of your forecasting requirements and whether the AI system can handle the intricacies of your data. A robust AI solution tailored to your business forecasting needs can provide more accurate predictions and insights, leading to better decision-making processes.
Moreover, it is crucial to evaluate the scalability and flexibility of the AI forecasting solution. Ensure that the chosen system can grow with your business and adapt to changing data dynamics. A customizable AI platform that can evolve alongside your business requirements is vital for long-term success in forecasting. By selecting a solution that aligns with your goals and can accommodate future growth, you can harness the full potential of AI technology for enhanced forecasting accuracy and efficiency.
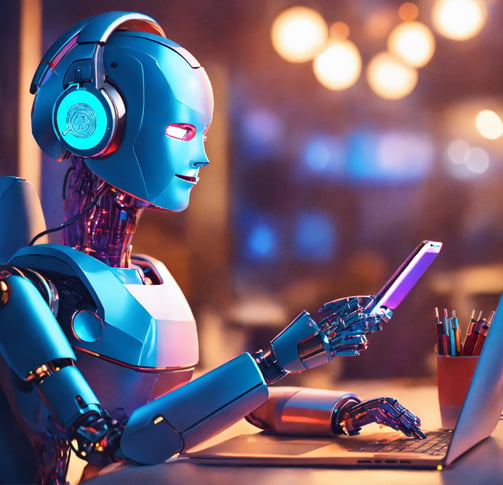
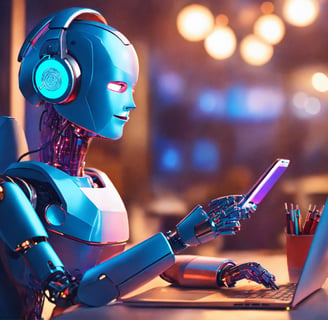
Future Trends in AI-Powered Forecasting
Continual advancements in forecasting technology are poised to revolutionize the predictive analytics landscape. As artificial intelligence becomes more sophisticated, the accuracy and reliability of forecasts are expected to reach unprecedented heights. These advancements will enable AI-powered forecasting systems to process and analyze vast amounts of data in real time, leading to more precise predictions and strategic insights for businesses.
Moreover, the integration of machine learning algorithms into forecasting models will allow for dynamic and adaptive forecasting approaches. This means that AI-powered forecasting systems will be able to learn from past data patterns and adjust their predictions accordingly, enhancing their predictive capabilities. As businesses increasingly rely on data-driven decision-making, these future trends in AI-powered forecasting are likely to play a pivotal role in driving strategic growth and competitive advantage.
What are some common challenges in traditional forecasting methods?
Common challenges in traditional forecasting methods include limited ability to handle large and complex datasets, insufficient accuracy in predicting future trends, and difficulty in adapting to changing market conditions.
How does AI-powered forecasting improve accuracy in predictions?
AI-powered forecasting utilizes advanced algorithms and machine learning techniques to analyze large amounts of data quickly and efficiently. This leads to more accurate predictions based on patterns and trends identified by the AI system.
What role does data play in AI-powered forecasting?
Data plays a crucial role in AI-powered forecasting as the accuracy of predictions depends on the quality and quantity of data available. AI systems use historical data to identify patterns and trends, which are then used to make predictions about future outcomes.
How can real-time data analysis benefit forecasting?
Real-time data analysis allows for up-to-date information to be incorporated into the forecasting process, leading to more accurate and timely predictions. This can help businesses make informed decisions quickly in response to changing market conditions.
What are some key considerations for businesses looking to implement AI-powered forecasting?
Some key considerations for businesses looking to implement AI-powered forecasting include evaluating the scalability and flexibility of the AI solution, ensuring compatibility with existing systems, and considering the level of technical expertise required for implementation and maintenance.
About the Author:
Waqar Jadoon, with 28 years in aviation supply chain management, holds an MBA and a software engineering degree. Renowned for streamlining operations and integrating AI and data analytics, he blends technical and business expertise to drive industry innovation. Waqar champions sustainable practices and technological advancements, shaping the future of aviation logistics