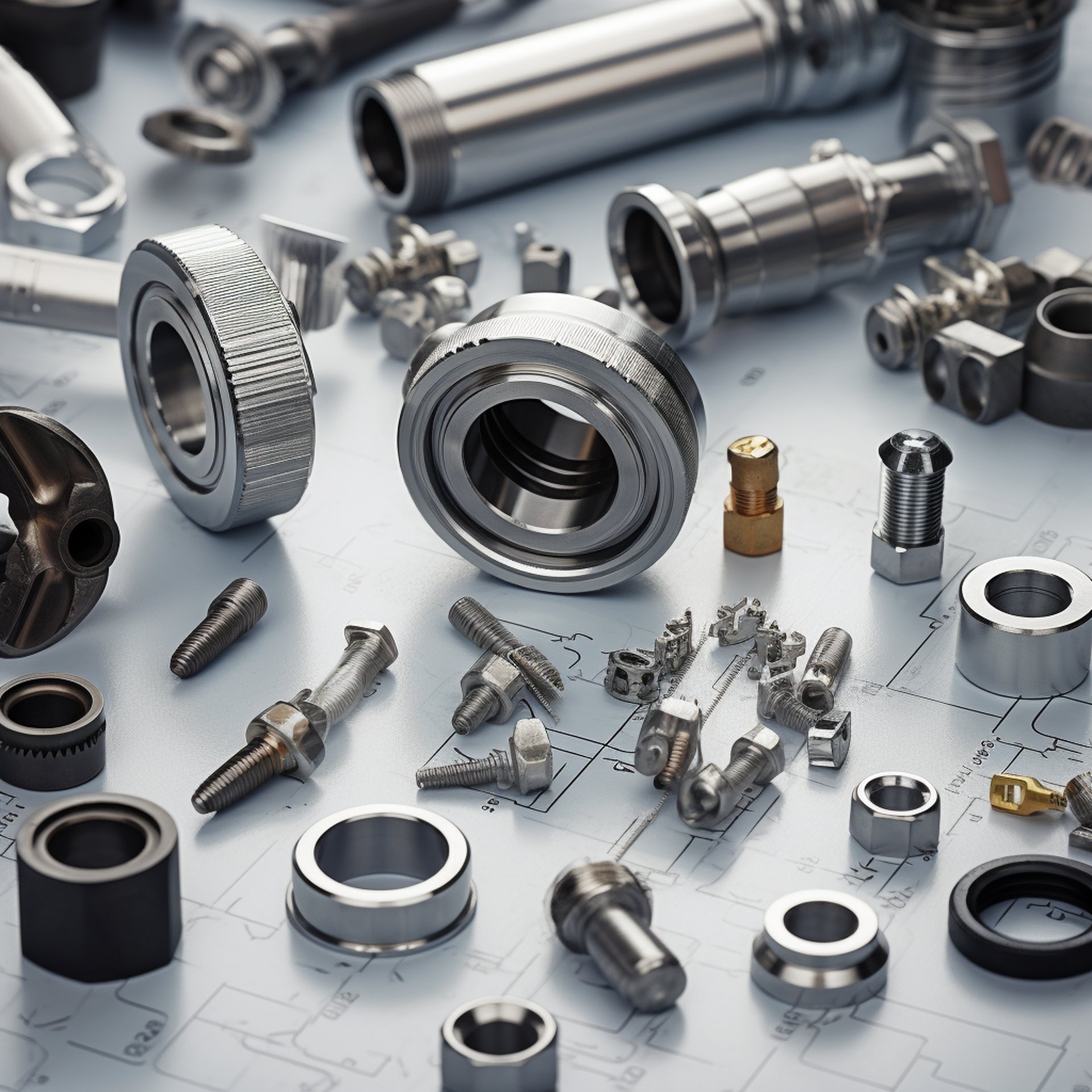
Don't Get Grounded by Guesswork: Embrace AI for Future-Proof Demand Forecasting in Aviation
Discover how embracing AI can revolutionize demand forecasting in the aviation industry. Don't rely on guesswork anymore; leverage advanced technologies to future-proof your business and make accurate predictions. Learn why AI-powered solutions are crucial for staying ahead of the competition, optimizing operations, and maximizing profitability. Stay informed with our insightful blog post on this game-changing topic.
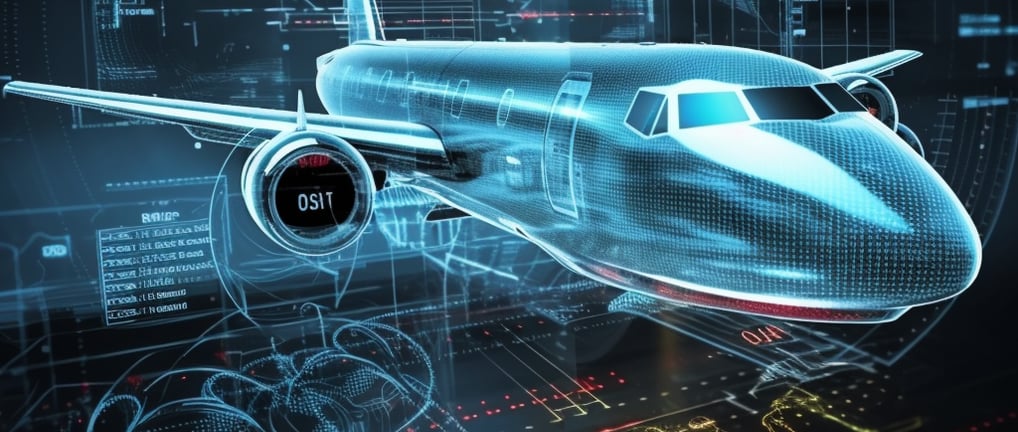
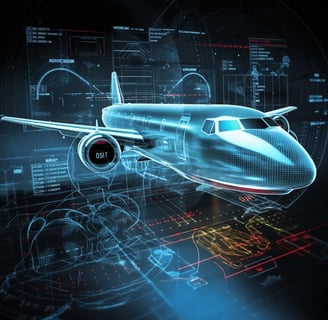
The Importance of Accurate Demand Forecasting in the Aviation Industry
Accurate demand forecasting plays a vital role in the aviation industry, as it enables airlines to optimize their operations and maximize profitability. With the ever-changing dynamics of the aviation industry, it becomes crucial for airlines to have a clear understanding of the market demand and customer preferences. By leveraging advanced techniques and technologies in aviation demand forecasting, airlines can align their strategic and operational decisions to cater to the evolving needs of the industry and gain a competitive edge.
Aviation demand forecasting allows airlines to effectively plan their capacity, route optimization, and inventory management, leading to cost savings and increased operational efficiency. Proper forecasting helps airlines to determine the right number of aircraft to deploy on specific routes, ensuring that there is neither overcapacity nor an insufficient number of seats to meet customer demands. Moreover, accurate demand forecasting helps airlines to adjust pricing strategies, flight schedules, and fleet planning, enabling them to offer competitive fares and improve customer satisfaction. By anticipating the changing trends and preferences of travelers, airlines can increase their revenue and maintain a loyal customer base.
The Challenges of Traditional Demand Forecasting Methods
The traditional demand forecasting methods in the aviation industry face numerous challenges that hinder their accuracy and effectiveness. Firstly, these methods often rely heavily on historical data and trends, which may not accurately reflect the ever-changing dynamics of the aviation market. As a result, traditional methods may fail to account for emerging patterns and disruptions, leading to inaccurate forecasts.
Secondly, traditional demand forecasting methods typically lack the capability to process and analyze vast amounts of data in real-time. This limitation makes it difficult to capture the full picture of customer preferences, market trends, and other relevant factors that influence demand. However, with the advent of artificial intelligence (AI) in aviation, these challenges are being addressed. AI-powered demand forecasting models can efficiently process and analyze large volumes of data from various sources, providing a more accurate and timely assessment of demand patterns. By incorporating machine learning algorithms, AI can continuously learn from new data and adapt its forecasting techniques accordingly, leading to improved accuracy and better decision-making from a strategic, operational, and trending perspective.
How Artificial Intelligence is Revolutionizing Demand Forecasting in Aviation
Artificial Intelligence (AI) has become an integral part of demand forecasting in the aviation industry, revolutionizing the way airlines predict customer demand for flights. With traditional methods often marred by inaccuracies and limitations, the incorporation of AI brings a new level of sophistication and efficiency to this critical aspect of operations. By leveraging advanced algorithms and machine learning techniques, AI enables airlines to predict demand with greater precision, helping them optimize resource planning, improve customer satisfaction, and maximize profitability.
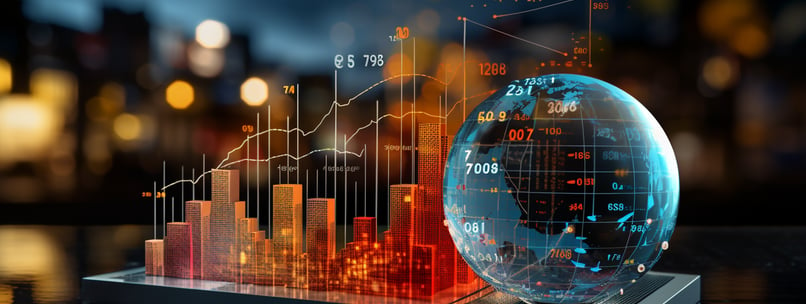
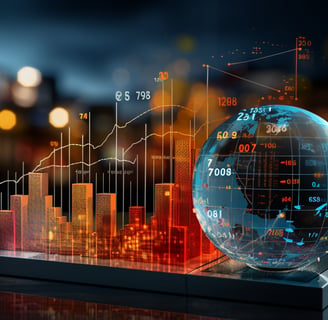
One of the key advantages offered by AI in demand forecasting is its ability to process large volumes of data quickly and accurately. AI algorithms can analyze historical travel patterns, customer preferences, weather conditions, and other relevant factors to generate accurate demand predictions. This allows airlines to make informed decisions at a strategic, operational, and even trending perspective. By identifying demand patterns and trends, airlines can adjust their flight schedules, seat capacities, and pricing strategies in real-time, ensuring they meet customer demands while avoiding under- or over-capacity scenarios. With the use of charts, graphs, references, and facts, airlines can visualize and present these demand predictions in a clear and concise manner, facilitating data-driven decision-making and enhancing overall operational efficiency.
Exploring the Role of Machine Learning in Demand Forecasting
Machine learning has emerged as a game-changing technology in demand forecasting, offering remarkable improvements in accuracy and efficiency. In the context of aviation, machine learning algorithms analyze massive amounts of historical data on flight bookings, passenger demographics, and travel patterns, providing valuable insights for inventory management. By learning from past trends and patterns, these algorithms can predict future demand with a high level of precision. This enables airlines to optimize their inventory, ensuring they have the right number of seats available on each flight to maximize revenue, minimize costs, and meet passenger expectations. Moreover, machine learning models can adapt and refine their predictions in real-time, taking into account factors such as changing market conditions or unforeseen events, providing airlines with a competitive advantage from a strategic, operational, and trending perspective.
Implementing machine learning in demand forecasting requires a structured approach and robust data infrastructure. Airlines need to invest in the collection, cleaning, and organization of data from various sources to train their machine learning models effectively. Historical data, flight schedules, weather conditions, customer profiles, and external factors such as holidays or events are among the many variables that need to be considered to build accurate forecasting models. Additionally, the integration of machine learning algorithms with inventory management systems is crucial for seamless decision-making and optimization. By combining the power of machine learning with the expertise of analysts, airlines can leverage advanced predictive analytics to make informed decisions, allocating resources, and managing inventory effectively.
In conclusion, machine learning plays a pivotal role in demand forecasting within the aviation industry. Its ability to analyze vast amounts of data and make accurate predictions offers immense value from a strategic, operational, and trending perspective. By embracing this technology, airlines can enhance their inventory management strategies, ensuring they meet passenger demand, optimize revenue, and stay competitive in an ever-evolving market. However, it is important for airlines to recognize the complexity of implementing machine learning and invest in the necessary resources to build robust forecasting models.
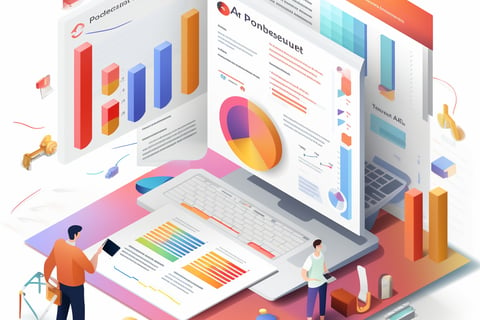
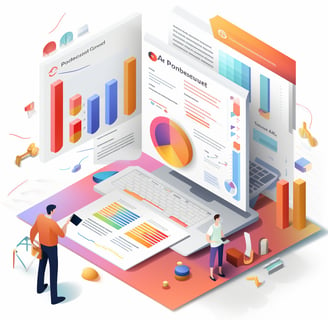
Leveraging Big Data for More Accurate Aviation Demand Forecasting
In the ever-evolving aviation industry, accurate demand forecasting is crucial for airlines to optimize costs and maximize profitability. Traditional methods of demand forecasting often fall short, relying on historical data and basic mathematical models. However, with the advent of big data, airlines now have the opportunity to leverage vast amounts of information to gain deeper insights into passenger behavior and market trends.
By harnessing big data, airlines can enhance their demand forecasting models from a strategic, operational, and trending perspective. Big data provides a comprehensive view of passenger preferences, allowing airlines to tailor their services and offerings accordingly. This can lead to improved customer satisfaction and loyalty, ultimately driving revenue growth. Moreover, big data enables airlines to identify patterns and trends in passenger demand, helping them anticipate fluctuations and make informed decisions about capacity planning, route optimization, and pricing strategies.
One of the key benefits of leveraging big data for demand forecasting is cost optimization. By analyzing historical data alongside external factors such as weather patterns, economic indicators, and even social media trends, airlines can accurately predict future demand and adjust their operations accordingly. This enables them to optimize fleet utilization, reduce fuel consumption, and streamline operational efficiency, leading to significant cost savings. Furthermore, big data analytics can help identify cost-saving opportunities across the entire supply chain, from procurement and maintenance to crew scheduling and airport operations.
In conclusion, leveraging big data for more accurate aviation demand forecasting brings numerous benefits to airlines, including cost optimization, improved customer satisfaction, and better decision-making. By embracing the power of big data analytics, airlines can gain a competitive edge in an industry where every percentage point of efficiency and accuracy matters. As the aviation industry continues to evolve, harnessing the potential of big data will become increasingly critical for airlines to thrive in a fast-paced and ever-changing market.
The Benefits of Embracing AI for Future-Proof Demand Forecasting
The aviation industry is constantly evolving, and demand forecasting plays a crucial role in its growth and success. Embracing artificial intelligence (AI) for future-proof demand forecasting offers numerous benefits that can propel airlines to new heights.
One of the key advantages of AI in demand forecasting is its ability to uncover growth potential. By analyzing vast amounts of data from various sources, AI algorithms can identify patterns, trends, and emerging markets that may otherwise go unnoticed. This enables airlines and airports to make strategic decisions based on a deep understanding of market dynamics, ensuring that they are well-prepared to capitalize on new opportunities for expansion. By leveraging AI, the aviation industry can proactively adapt to changing consumer preferences and effectively allocate resources to drive sustainable growth.
From an operational perspective, embracing AI in demand forecasting can optimize airline operations and enhance efficiency. AI algorithms can analyze historical data, weather conditions, competitor behavior, and other relevant factors to provide accurate demand predictions. This allows airlines to optimize flight schedules, crew allocation, and inventory management, minimizing costs and maximizing revenue. By automating these processes and relying on AI-driven insights, airlines can streamline operations, improve on-time performance, and deliver an enhanced customer experience. The benefits of AI in future-proof demand forecasting extend beyond the immediate operational gains, empowering airlines to make informed decisions that drive long-term profitability and sustained success.
Case Study: Systematic review of passenger demand forecasting in aviation industry
Forecasting aviation demand is a significant challenge in the airline industry. The design of commercial aviation networks heavily relies on reliable travel demand predictions. It enables the aviation industry to plan ahead of time, evaluate whether an existing strategy needs to be revised, and prepare for new demands and challenges. This study examines recently published aviation demand studies and evaluates them in terms of the various forecasting techniques used, as well as the advantages and disadvantages of each. This study investigates numerous forecasting techniques for passenger demand, emphasizing the multiple factors that influence aviation demand. It examined the benefits and drawbacks of various models ranging from econometric to statistical, machine learning to deep neural networks, and the most recent hybrid models. This paper discusses multiple application areas where passenger demand forecasting is used effectively. In addition to the benefits, the challenges and potential future scope of passenger demand forecasting were discussed. This study will be helpful to future aviation researchers while also inspiring young researchers to pursue careers in this industry.
Read more @: https://link.springer.com/article/10.1007/s11042-023-15552-1
Overcoming Resistance to AI Adoption in Demand Forecasting
Overcoming resistance to AI adoption in demand forecasting is crucial for the aviation industry to harness the full potential of this transformative technology. While AI offers significant benefits, such as improved accuracy and efficiency in demand forecasting, some professionals may be hesitant to embrace it due to concerns about job displacement or the complexity of implementation. However, it is essential to approach this challenge with resilience and recognize the long-term advantages that AI can bring.
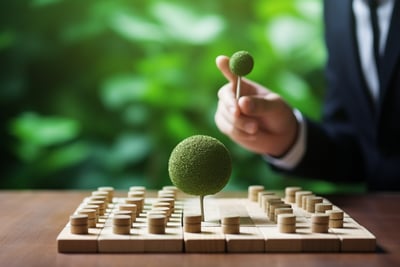
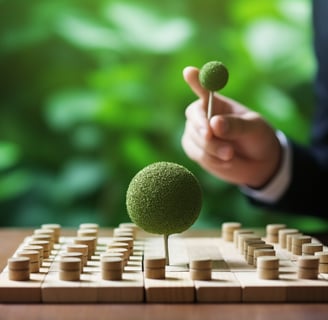
To address the resistance to AI adoption, organizations should focus on comprehensive change management strategies. By communicating the strategic rationale behind integrating AI into demand forecasting processes and highlighting the operational efficiencies it can unlock, stakeholders can better understand the value proposition. Additionally, sharing success stories and case studies from early adopters in the industry can provide tangible evidence of AI's positive impact in demand forecasting. Moreover, gathering and presenting quantitative evidence, such as charts and graphs showcasing the accuracy improvements achieved through AI implementations, can further substantiate the benefits. Collaborating with experts and leveraging industry research and trends can help build a persuasive argument for AI adoption in demand forecasting and alleviate concerns.
The Future of Demand Forecasting in the Aviation Industry
The future of demand forecasting in the aviation industry is poised for significant advancements as organizations increasingly recognize the value of data-driven insights. With the advent of artificial intelligence (AI) and machine learning (ML) technologies, aviation companies can now leverage vast amounts of data to gain a deeper understanding of customer behavior, market trends, and operational dynamics. By harnessing the power of these technologies, organizations can make more informed decisions, optimize their resources, and improve customer satisfaction.
One of the key benefits of data-driven insights in demand forecasting is the ability to identify emerging trends and patterns that may have been overlooked using traditional methods. By analyzing historical data alongside real-time information, aviation companies can gain a comprehensive view of the market and adapt their strategies accordingly. With the help of AI and ML algorithms, these organizations can identify factors that influence demand, such as seasonality, price fluctuations, and customer preferences, allowing them to make data-backed predictions and plan their operations more effectively.
In conclusion, the future of demand forecasting in the aviation industry is poised to undergo a transformational shift, thanks to the adoption of AI and ML technologies. By harnessing the power of data-driven insights, organizations can gain a competitive edge by making more informed decisions and improving their forecasting accuracy. As the industry continues to evolve, it is imperative for aviation companies to embrace these advancements to stay ahead of the curve and maximize their operational efficiency.
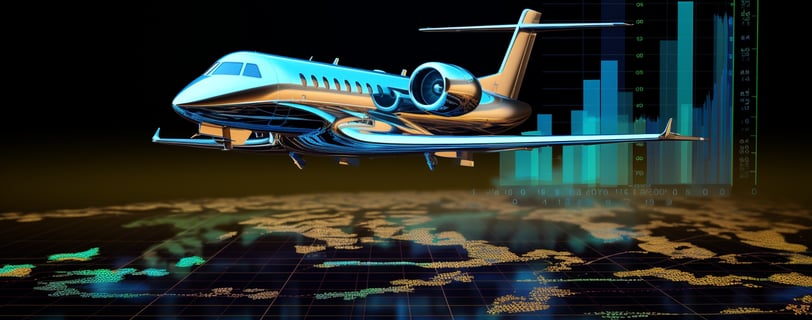
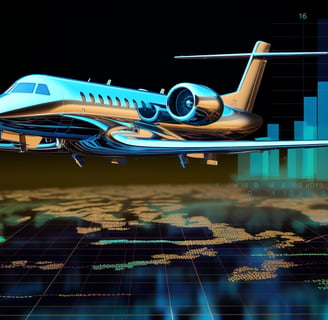
Best Practices for Implementing AI in Aviation Demand Forecasting
Best Practices for Implementing AI in Aviation Demand Forecasting
Implementing artificial intelligence (AI) in aviation demand forecasting requires a strategic and operational approach to ensure its successful integration. One of the best practices is to have a well-defined goal and objective for adopting AI in demand forecasting. This involves identifying the specific challenges and areas where AI can add value, such as improving accuracy, speed, and efficiency. By setting clear goals, airlines and aviation companies can align their efforts and resources towards achieving tangible results.
Another important best practice is to invest in data quality and infrastructure. AI algorithms heavily rely on high-quality data to generate accurate and reliable forecasts. Therefore, it is crucial to ensure that the necessary data sources are in place and that the data is complete, consistent, and up-to-date. Additionally, having a robust infrastructure that can handle large volumes of data and support AI-driven analytics is essential for successful implementation. This may involve upgrading existing systems or investing in new technologies to support the AI capabilities.
Overall, in order to implement AI in aviation demand forecasting successfully, it is vital to adopt a strategic and operational perspective. By setting clear goals and investing in data quality and infrastructure, airlines and aviation companies can leverage the power of AI to improve their forecasting accuracy and make more informed business decisions. This not only helps in meeting customer demand but also enhances operational efficiency and ultimately contributes to the success of the aviation industry as a whole.
Featured Questions:
Why is accurate demand forecasting important in the aviation industry?
Accurate demand forecasting is crucial in the aviation industry as it helps airlines and airports optimize their resources, make informed decisions, improve customer satisfaction, and increase profitability.
What are the challenges of traditional demand forecasting methods in aviation?
Traditional demand forecasting methods in aviation often rely on historical data and subjective human judgment, which can be limited in accuracy and unable to adapt to dynamic market conditions. Additionally, they may not effectively incorporate diverse factors that influence demand, such as weather patterns or social trends.
How is artificial intelligence revolutionizing demand forecasting in aviation?
Artificial intelligence is revolutionizing demand forecasting in aviation by utilizing advanced algorithms and machine learning techniques to analyze vast amounts of data, identify patterns and trends, and generate more accurate predictions. It enables real-time and automated decision-making, improving the efficiency and effectiveness of forecasting processes.
What is the role of machine learning in demand forecasting?
Machine learning plays a crucial role in demand forecasting by employing algorithms that can learn from historical data, identify patterns, and make predictions based on relevant variables. It enables the automation of forecasting models, leading to more accurate and timely predictions in the aviation industry.
How can big data be leveraged for more accurate aviation demand forecasting?
Big data can be leveraged in aviation demand forecasting by integrating various data sources, such as passenger booking information, flight schedules, weather data, social media feeds, and economic indicators. Analyzing these vast datasets using AI techniques allows for a more comprehensive understanding of demand drivers and enhances the accuracy of forecasting models.
What are the benefits of embracing AI for future-proof demand forecasting in aviation?
Embracing AI for demand forecasting in aviation brings several benefits, including improved accuracy, enhanced operational efficiency, reduced costs, better resource allocation, increased customer satisfaction, and the ability to adapt to changing market dynamics. It enables the industry to stay competitive and future-proof its forecasting capabilities.
Can you provide any case studies of successful AI implementation in aviation demand forecasting?
Yes, case studies of successful AI implementation in aviation demand forecasting demonstrate how airlines and airports have achieved significant improvements in forecasting accuracy, optimized scheduling, and improved revenue management. These case studies highlight the tangible benefits of adopting AI in this domain.
How can resistance to AI adoption in demand forecasting be overcome?
Resistance to AI adoption in demand forecasting can be overcome by providing comprehensive training and education on AI benefits, addressing concerns about job displacement, showcasing successful case studies, emphasizing the collaboration between AI and human expertise, and fostering a culture of innovation and openness to change.
What does the future hold for demand forecasting in the aviation industry?
The future of demand forecasting in the aviation industry is likely to be increasingly driven by AI and machine learning technologies. It will involve more real-time data integration, advanced predictive analytics, and the continuous refinement of forecasting models to meet evolving market demands and optimize resource allocation.
What are the best practices for implementing AI in aviation demand forecasting?
The best practices for implementing AI in aviation demand forecasting include selecting high-quality data sources, leveraging advanced analytics tools, building robust and scalable AI models, collaborating across organizational functions, continuously monitoring and updating models, and fostering a culture of data-driven decision-making.
About the Author:
Waqar Jadoon, with 28 years in aviation supply chain management, holds an MBA and a software engineering degree. Renowned for streamlining operations and integrating AI and data analytics, he blends technical and business expertise to drive industry innovation. Waqar champions sustainable practices and technological advancements, shaping the future of aviation logistics.

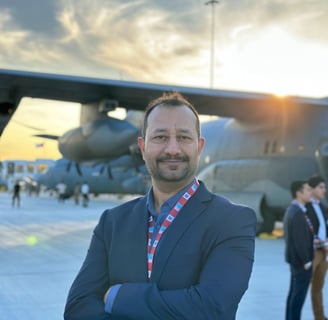