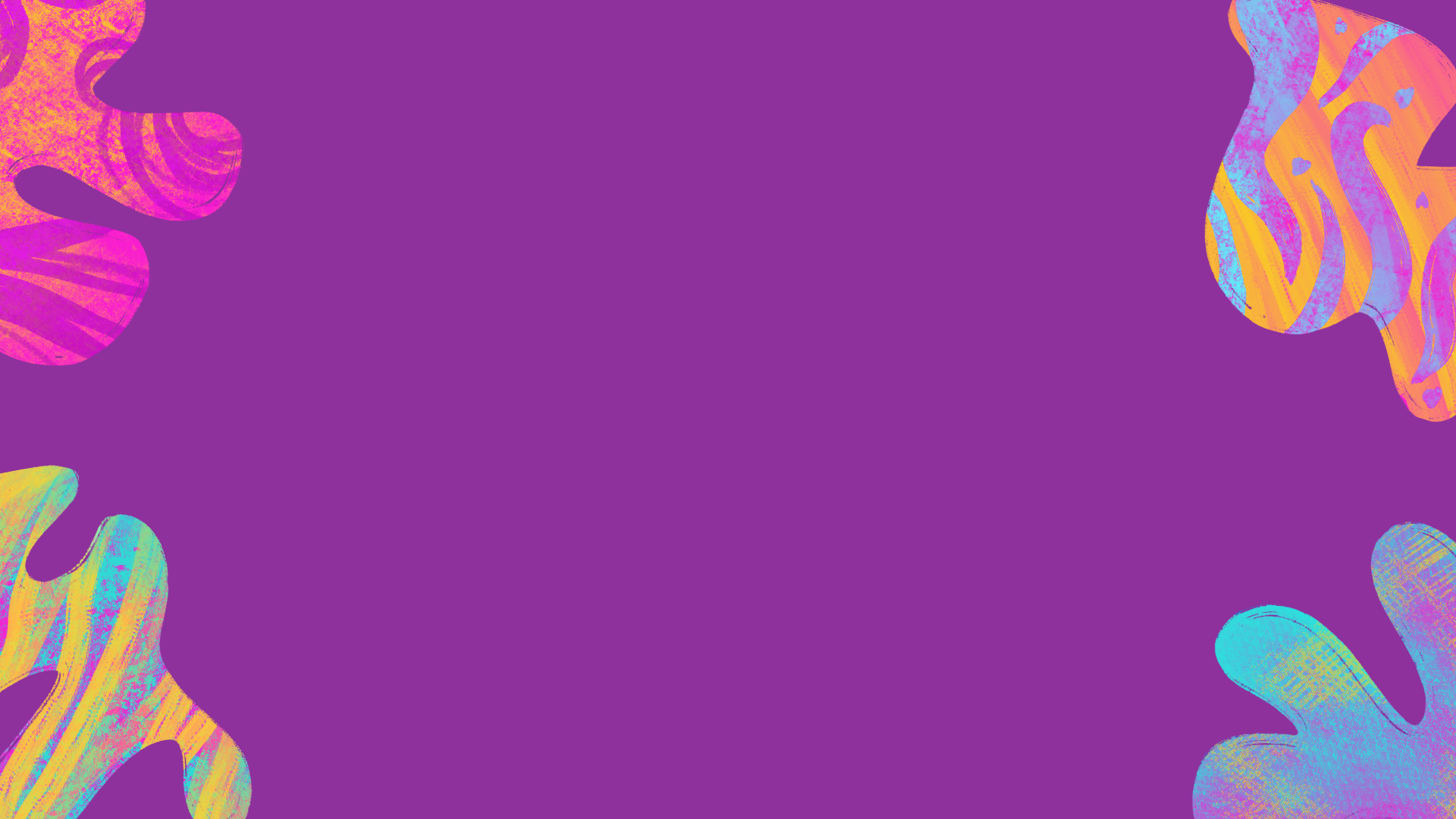
Boosting Demand Forecasting Accuracy: How to Leverage Real-Time Sales Data for Precise Predictions
Learn how to improve demand forecasting accuracy by leveraging real-time sales data in this insightful blog post. Discover the strategies and techniques that can help you make more precise predictions for your business.
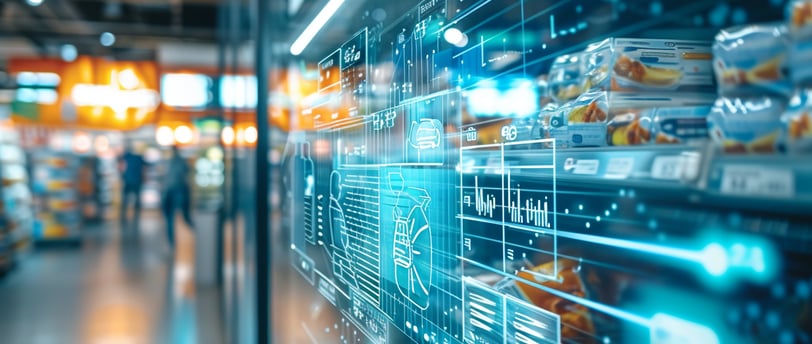
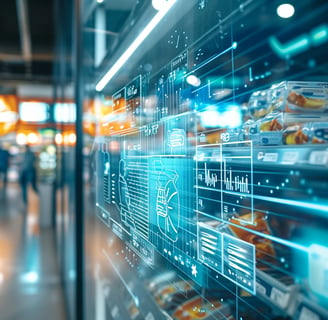
The Importance of Demand Forecasting Accuracy
Demand forecasting accuracy is a critical aspect of any successful business operation. Without precise predictions of future demand for products or services, companies may face a number of challenges such as excess inventory, stockouts, or missed revenue opportunities. In today's competitive marketplace, where consumer preferences are constantly evolving, the ability to forecast demand with accuracy is more crucial than ever for maintaining a competitive edge.
Ensuring high demand forecasting accuracy allows businesses to optimize their inventory levels, streamline production processes, and enhance overall operational efficiency. By leveraging accurate demand forecasts, organizations can make informed decisions regarding resource allocation, pricing strategies, and market positioning. Ultimately, the ability to anticipate and respond to fluctuations in demand with precision is a key driver of sustained business success in an ever-changing economic landscape.
Understanding the Role of Real-Time Sales Data
Real-time sales data plays a pivotal role in shaping a company's demand forecasting strategies. By providing up-to-the-minute insights into customer preferences and market trends, real-time sales data enables businesses to make informed decisions promptly. This type of data empowers organizations to adapt swiftly to changing consumer behavior and market conditions, ensuring that inventory levels are optimized and customer demand is met efficiently.
The utilization of real-time sales data allows businesses to track sales performance instantaneously, identifying patterns and trends that can guide strategic planning and resource allocation. By harnessing the power of this data, companies can operate with agility and stay ahead of the competition in a dynamic marketplace. Real-time sales data serves as a valuable asset in enhancing operational efficiency and driving revenue growth, positioning businesses for success in an ever-evolving business landscape.
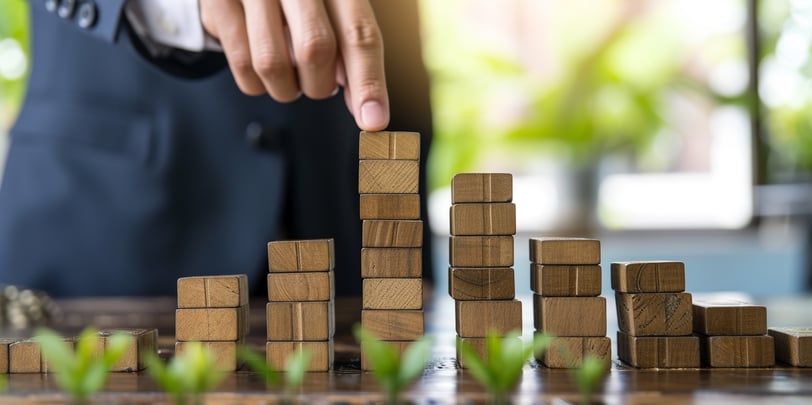
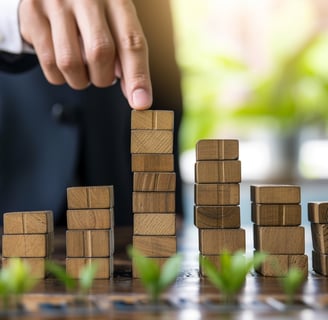
Key Challenges in Demand Forecasting
One of the primary challenges in demand forecasting lies in the complexity of predictive analytics. Companies often struggle with analyses that require intricate algorithms to accurately predict future demand patterns. Incorporating advanced statistical techniques to comprehend market trends further adds to the complexity, making it crucial for organizations to invest in skilled data scientists who can navigate through these complexities.
Another key challenge arises from the volatility of external factors impacting demand forecasting. External variables such as economic conditions, competitor actions, and changing consumer preferences introduce uncertainties that can significantly affect the accuracy of predictions. This unpredictability calls for a proactive approach in constantly monitoring and adjusting predictive models to minimize the margin of error, ensuring that businesses remain agile in responding to market dynamics.
Benefits of Leveraging Real-Time Sales Data
In the realm of sales forecasting techniques, the incorporation of real-time sales data holds immense benefits for businesses striving to stay ahead in today's dynamic market landscape. Leveraging real-time sales data enables organizations to gain a comprehensive understanding of their current performance metrics and consumer behavior patterns. This invaluable insight empowers decision-makers to promptly adjust strategies, anticipate demand fluctuations, and capitalize on emerging trends, fostering a more agile and responsive operational framework.
Moreover, the utilization of real-time sales data significantly enhances the precision and reliability of sales forecasting techniques. By integrating up-to-the-minute sales information, businesses can achieve more accurate predictions regarding future demand, inventory requirements, and revenue projections. This heightened accuracy enables companies to streamline inventory management, minimize stockouts or overstock situations, optimize pricing strategies, and ultimately boost overall operational efficiency and profitability.
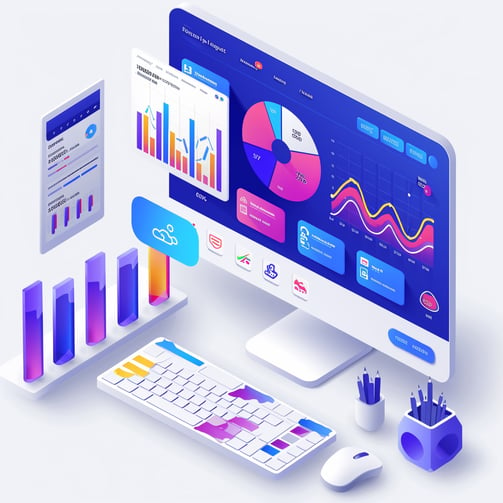
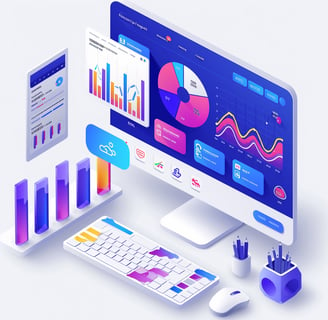
Best Practices for Integrating Real-Time Sales Data
When it comes to integrating real-time sales data into demand forecasting strategies, organizations must prioritize data-driven forecasting methods to enhance accuracy and agility in decision-making processes. By leveraging real-time sales data effectively, businesses can gain valuable insights into current market trends and customer behaviors, enabling them to adjust their inventory levels and production schedules with greater precision.
One of the key best practices for integrating real-time sales data is to establish automated processes that capture and analyze data in real time. This ensures that businesses have access to up-to-date information that can inform their forecasting models and strategic planning. By utilizing advanced analytics tools and machine learning algorithms, organizations can extract meaningful patterns and correlations from real-time sales data, enabling them to make data-driven decisions that drive business growth and competitiveness in today's dynamic market landscape.
Utilizing Historical Data for More Accurate Predictions
In the realm of demand forecasting accuracy, the utilization of historical data plays a pivotal role in enhancing the precision of predictions. By delving into past market trends and consumer behaviors, businesses can conduct thorough market demand analysis to extract valuable insights for making informed decisions. Such an approach not only aids in understanding the dynamics of the market but also enables organizations to anticipate future fluctuations with greater confidence.
When it comes to leveraging historical data for more accurate predictions, it is imperative for businesses to adopt a structured approach that entails meticulous data collection and comprehensive analysis. By scrutinizing past sales figures, seasonal patterns, and other relevant data points, companies can discern patterns and trends that offer valuable clues about future demand fluctuations. By incorporating historical data into their forecasting models, organizations can establish a solid foundation for making data-driven decisions that are in alignment with market realities and consumer preferences.
Implementing Advanced Analytics for Precise Forecasting
Forecasting demand accurately is essential for businesses to optimize their inventory levels and meet customer needs efficiently. Implementing advanced analytics tools allows companies to delve deeper into sales data, trends, and patterns to make more precise predictions. By leveraging these analytics, organizations can identify key drivers affecting demand, enabling them to adjust their inventory levels accordingly for optimal inventory optimization.
Furthermore, advanced analytics for precise forecasting not only aids in inventory optimization but also in streamlining supply chain operations. By utilizing predictive modeling and data-driven insights, businesses can better anticipate future demand fluctuations and proactively plan for inventory management. This proactive approach not only ensures product availability but also helps in reducing excess inventory costs and mitigating stockouts, ultimately leading to improved operational efficiency and customer satisfaction.
The Role of Machine Learning in Demand Forecasting
In modern business environments, the utilization of machine learning algorithms has revolutionized demand forecasting practices. Harnessing the power of artificial intelligence, machine learning algorithms are capable of processing vast amounts of data to generate precise and actionable insights. These advanced analytical tools enable organizations to predict future demand patterns with a higher degree of accuracy, facilitating strategic decision-making and enhancing operational efficiency.
As the cornerstone of business intelligence, machine learning in demand forecasting empowers enterprises to unlock valuable insights from complex datasets. By leveraging sophisticated algorithms, organizations can uncover hidden trends, patterns, and correlations that may not be discernible through traditional forecasting methods. Additionally, machine learning models can adapt and self-improve over time, continuously refining their predictive capabilities to deliver more reliable forecasts.
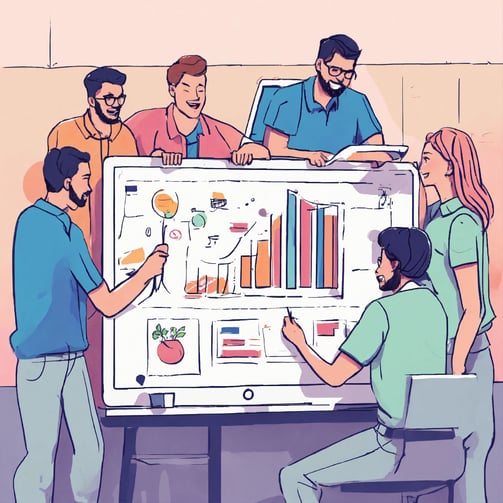
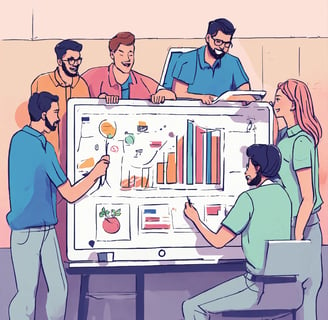
Measuring the Success of Demand Forecasting Strategies
Sales data analysis is paramount in evaluating the effectiveness of demand forecasting strategies. By assessing the accuracy of predictions against the actual sales figures, organizations can gauge the reliability of their forecasting models. This analysis provides valuable insights into the strengths and weaknesses of the forecasting process, helping businesses refine their approaches and make more informed decisions regarding inventory management and resource allocation.
Furthermore, sales data analysis allows companies to track the performance of different product lines, identify trends, and detect potential anomalies. By comparing the forecasted demand with the actual sales data, organizations can measure the precision of their forecasting methods and make necessary adjustments to enhance future predictions. Through continuous monitoring and assessment of demand forecasting strategies using sales data analysis, businesses can optimize their operations and improve overall efficiency in meeting customer needs.
Continuous Improvement in Demand Forecasting Accuracy
To achieve long-term success in demand forecasting, companies must prioritize continuous improvement in forecast accuracy. This involves a systematic approach to analyzing past forecasting errors, identifying patterns, and implementing adjustments to enhance predictive capabilities. By consistently refining forecasting models and methodologies, organizations can stay ahead of market fluctuations and better align supply chain operations with actual customer demand.
Sustained forecast accuracy improvement requires a commitment to ongoing data analysis and collaboration across departments. Regular evaluations of forecasting performance metrics, such as forecast error rates and bias, can pinpoint areas for enhancement and guide future forecasting strategies. Additionally, incorporating feedback from frontline sales teams and incorporating market intelligence into forecasting algorithms can further refine predictions and optimize inventory management decisions.
Why is demand forecasting accuracy important?
Demand forecasting accuracy is crucial for businesses to effectively manage their inventory levels, production schedules, and overall supply chain operations. Accurate forecasting helps reduce costs, minimize stockouts, and improve customer satisfaction.
How can real-time sales data improve demand forecasting accuracy?
Real-time sales data provides up-to-date information on consumer behavior, market trends, and other factors that can impact demand. By incorporating this data into forecasting models, businesses can make more informed decisions and respond quickly to changing market conditions.
What are some key challenges in demand forecasting?
Some key challenges in demand forecasting include seasonality, fluctuations in consumer demand, inaccurate historical data, and external factors such as economic conditions or competitor actions. Overcoming these challenges requires a combination of data analysis, predictive modeling, and strategic planning.
How can businesses benefit from leveraging real-time sales data?
By leveraging real-time sales data, businesses can improve demand forecasting accuracy, optimize inventory levels, identify potential sales opportunities, and enhance overall operational efficiency. This can lead to increased revenue, reduced costs, and improved customer satisfaction.
What are some best practices for integrating real-time sales data into demand forecasting?
Some best practices for integrating real-time sales data include automating data collection processes, using advanced analytics tools, collaborating cross-functionally within the organization, and continuously evaluating and adjusting forecasting models based on new data.
How important is historical data for accurate demand forecasting?
Historical data plays a critical role in demand forecasting as it provides insights into past trends, patterns, and seasonal variations in demand. By analyzing historical data, businesses can make more informed predictions about future demand and adjust their strategies accordingly.
What is the role of machine learning in demand forecasting?
Machine learning algorithms can analyze large volumes of data, identify patterns, and make accurate predictions based on historical trends and real-time information. By leveraging machine learning techniques, businesses can enhance the precision and accuracy of their demand forecasting models.
How can businesses measure the success of their demand forecasting strategies?
Businesses can measure the success of their demand forecasting strategies by comparing forecasted demand with actual sales data, tracking key performance indicators (KPIs) such as forecast error rates and inventory turnover ratios, and soliciting feedback from stakeholders across the organization.
How can businesses continuously improve their demand forecasting accuracy?
Businesses can continuously improve their demand forecasting accuracy by regularly updating their data sources, refining forecasting models based on new information, investing in advanced analytics tools, and fostering a culture of data-driven decision-making within the organization
About the Author:
Waqar Jadoon, with 28 years in aviation supply chain management, holds an MBA and a software engineering degree. Renowned for streamlining operations and integrating AI and data analytics, he blends technical and business expertise to drive industry innovation. Waqar champions sustainable practices and technological advancements, shaping the future of aviation logistics